Machine learning builds models based on available sample data to make predictions and decisions on unexplored regions. In material science, machine learning has proved to have unprecedented abilities to find new stable materials, calculate numerous material properties, and speed up first-principle calculations. Our machine learning focuses on material component/structure discovery, material properties’ prediction, and physics-informed machine learning.
Computational physics studies various kinds of computational calculations, including
- first-principles DFT, effective medium theory, molecular dynamics, analytical modeling, and numerical simulations
- to predict materials properties and structures for 2D materials, interfaces and heterostructures;
- glass transition, flow of glassy polymer nanofilms; electron transport in solid-state quantum devices; formation of nanostructures in strained thin films;
- machine learning and high-throughput calculations; photonic crystals, topological photonics, plasmonic nanostructures, metamaterials, transformation optics, optomechanics of structured nanomaterials, and phononic crystals.
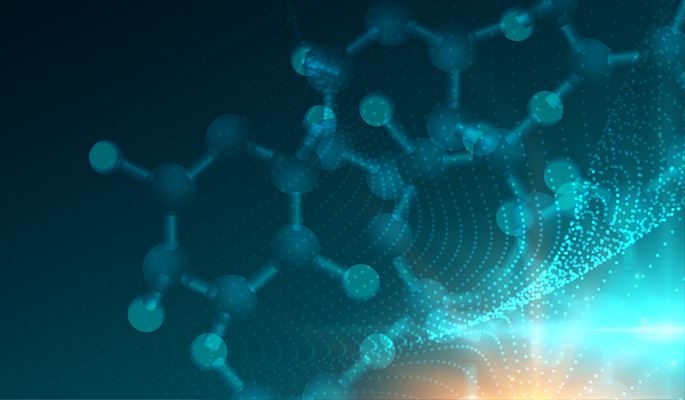