Non-invasive diagnostic device Smart-CKD for advancing clinical management of chronic kidney disease
Chronic kidney disease (CKD) affects 10% of the global population, among which renal fibrosis is a progressive process that can lead to end-stage renal failure. Early diagnosis and active monitoring are particularly important. Researchers from PolyU's Department of Health Technology and Informatics have developed Smart-CKD (S-CKD), a non-invasive computer-aided diagnostic tool. It integrates ultrasound data and selected clinical variables to provide clinical insights and assesses the risk of moderate-to-severe renal fibrosis progression in CKD patients with a promising diagnostic efficiency of 80%. The research findings have been published in the journal Academic Radiology.
Early diagnosis and accurate staging of renal fibrosis significantly guide treatment strategies and prognostic assessment, enabling timely preventive measures to avoid or delay disease exacerbations. However, identifying individuals at high risk of advanced renal fibrosis with precision continues to pose a challenge in clinical practice.
To tackle this challenge, the research team have collaborated with experts from The Fifth Affiliated Hospital of Sun Yat-sen University to invent S-CKD, which aims to improve disease progress monitoring and clinical management of CKD using informatics technology. This device offers a cost-effective solution for guiding patient management, thereby contributing notable clinical advantages.
While renal biopsy remains the gold standard for diagnosing and staging renal fibrosis, its invasive nature imposes limitations on conducting multiple observations and follow-ups, and has potential complications. Therefore, there is a pressing need to develop a non-invasive biomarker for precise monitoring and clinical management of renal fibrosis and its progression.
By leveraging machine learning, S-CKD integrates three pivotal clinical parameters - age, ultrasonic renal length and end-diastolic flow velocity of the interlobar renal artery, to assist medical practitioners in assessing renal fibrosis risk in CKD patients during routine clinical practices. It plays a crucial role in guiding treatment decisions and improving patient prognosis. S-CKD is accessible through an online web-based platform or in an offline document-based format, making it a user-friendly auxiliary instrument for flexible clinical applications.
The research on S-CKD titled “Development and Deployment of a Novel Diagnostic Tool Based on Conventional Ultrasound for Fibrosis Assessment in Chronic Kidney Disease” was published on Academic Radiology in September 2023. Research results showed S-CKD has excellent predictive accuracy and high clinical application value.
Moreover, the research team has developed a random forest (RF) model which combines 2-D shear wave elastography (SWE) data and clinical features of CKD patients for renal fibrosis evaluation. RF is a machine learning algorithm that generates a single result from multiple informative variables. The research titled “Assessment of Renal Fibrosis in Patients with Chronic Kidney Disease Using Shear Wave Elastography and Clinical Features: A Random Forest Approach” was published on Ultrasound in Medicine & Biology in July 2023.
The diagnostic field of renal fibrosis has seen remarkable progress with ultrasound elastography. However, this diagnostic modality highly depends on the operator’s experience and technical proficiency, which poses challenges for implementation, particularly in resource-limited areas. The primary aim of inventing S-CKD is to enable easy and ubiquitous applications in clinical management, even in the face of resource constraints. The input variables for S-CKD can be readily obtained from medical records and routine imaging assessment at a low cost, while the output probability can accurately and dynamically stratify patient risks. This established tool provides accurate information for patient-centered, informed decisions regarding the clinical management of CKD progression through active surveillance and non-invasive assessment.
For another ground-breaking innovation, the research team has introduced ultrasound radiomics analysis, advancing from clinical parameters to ultrasound images analysis. Radiomics, an emerging technology, enables the high-throughput extraction of numerous imaging features from medical imaging data that are invisible to the naked eye. This subsequently builds a radiomics model to achieve non-invasive assessment of renal fibrosis.
In particular, the team has applied radiomics technology to extract features from ultrasound images which may be incomprehensible and invisible to the naked eye. This is achieved by using data-characteristics algorithms based on machine learning. Additionally, this radiomics approach combines ultrasound images with clinical variables to construct a diagnostic model, which is subsequently visualised as a web-page calculator. The research titled “Ultrasound-based Radiomics Analysis in the Assessment of Renal Fibrosis in Patients with Chronic Kidney Disease,” was published on Abdominal Radiology in August 2023.
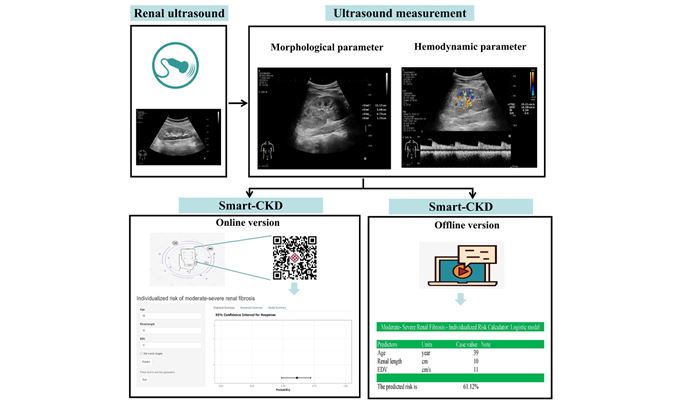