Machine Learning Design to Improve the Operational Efficiency of Transportation and Aviation
Seminar
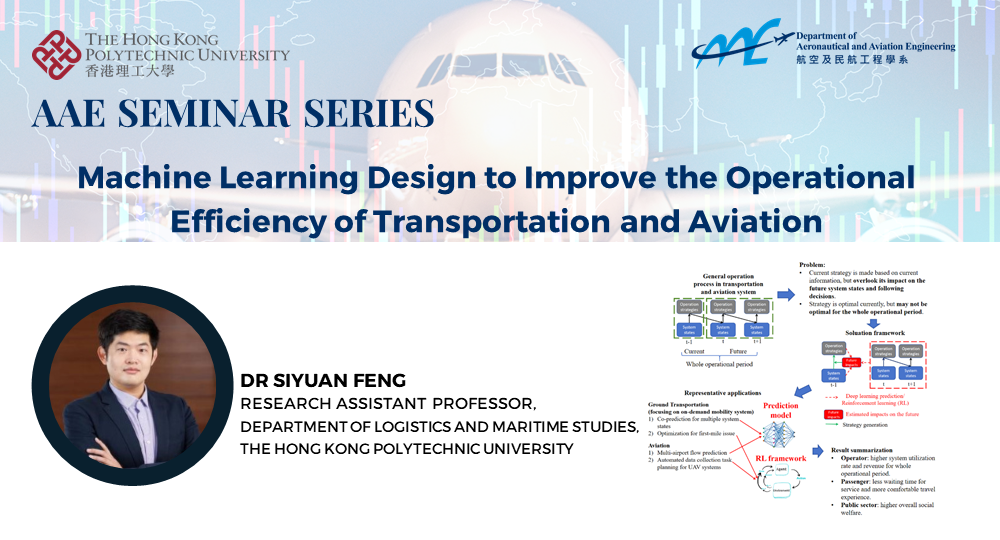
-
Date
09 Dec 2024
-
Organiser
Department of Aeronautical and Aviation Engineering
-
Time
14:30 - 15:30
-
Venue
FJ304 Map
Enquiry
General Office aae.info@polyu.edu.hk
Remarks
To receive a confirmation of attendance, please present your student or staff ID card at check-in.
Summary
Abstract
The ground transportation system (such as public transit and ride-sourcing mobility) and aviation systems (such as civil aviation and urban air mobility) together constitute the primary modes for people's daily short- and long-distance travel. Each transportation system has its unique operation process, and optimizing these operations to enhance the overall efficiency of transportation systems is a key concern for researchers and industry. By abstracting and generalizing different dynamic operations (as shown in the “General operation process” in the Fig.), we observe that there is often a continuous interaction between transportation systems and operational strategies. The result of current operational decisions can impact the future state of the system and subsequent decisions. In other words, if operational strategies are designed by considering only current and historical system information while ignoring their impact on future systems and strategies, the resulting decisions may be optimal in the short term but suboptimal over the entire operational cycle. To address the problem, we have considered two types of machine learning (ML) based solution frameworks. The first utilizes deep learning (DL) to accurately predict future system states and then integrates this predictive information into the current strategy design. The second employs reinforcement learning (RL) to directly generate farsighted strategies that benefit the entire operational cycle. The commonality between these approaches is their proactive use of future information to aid decision-making, thereby broadening the decision-making perspective. Additionally, both frameworks can rapidly extract information from the big data generated by the daily operations of transportation and aviation systems to optimize decisions. In this seminar, Dr Feng will focus on the application of these two frameworks to four critical operational issues in transportation and aviation systems, together with targeted machine learning model design and experimental validation. The results indicate that the respective models can effectively enhance the benefits for various stakeholders within the relevant systems (e.g., operators, passengers, public sectors). Finally, Dr Feng will discuss further directions and scenarios for the application of ML in transportation and aviation operation optimization.
Speaker
Dr Siyuan Feng serves as a Research Assistant Professor in the Department of Logistics and Maritime Studies at the Hong Kong Polytechnic University. He obtained his PhD degree in the Department of Civil and Environmental Engineering of The Hong Kong University of Science and Technology (with Hong Kong PhD Fellowship Scheme); his master degree in Civil Engineering of UC Berkeley and his bachelor degree in Civil Engineering of Tongji University. His research interests include machine learning for multi-modal transportation, deep learning based air transport management, reinforcement learning based unmanned aerial vehicle (UAV) fleet management and transportation simulation. He has published 13 papers in peer-reviewed high-quality journals such as Transportation Research (TR Part C/E) and IEEE Transactions on Intelligent Transportation Systems (IEEE TITS), and secured 2 research projects, including 1 General Research Fund (GRF) project and 1 collaborative research project with Didi Chuxing. He also obtained academic awards for his research, including HKUST SENG PhD Research Excellence Award Finalist (2023), Hong Kong Society for Transportation Studies (HKSTS) Outstanding Dissertation Award Honorable Mention (2024), and Young Scientist Awards Shortlist of Hong Kong Institution of Science (2024).