Interviews with Faculty Researchers

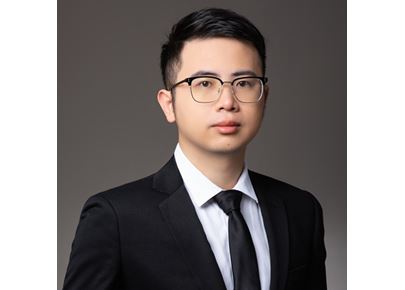
Emerging Computing Devices for Low-power Artificial Intelligence of Things
– Interview with Dr Chen Jiewei
Research Assistant Professor, Department of Applied Physics
As the demand for advanced sensing and computing devices in the Artificial Intelligence of Things (AIoT) grows, power consumption has become a major challenge. Traditional transistors use charge-based information carriers, which lead to significant energy waste from heat generation, failing to meet the rising energy demands of AI applications. To tackle this issue, Dr Chen Jiewei is developing next-generation transistors that aim to revolutionize modern electronics, by focusing on creating transistors from topological and two-dimensional materials that operate on different principles.
Dr Chen has developed topological phase change transistors using the Weyl semiconductor Tellurium (Te). The Te channel switches between topological phases via electrostatic modulation. It turns OFF with low conductance when the Fermi level is in the band gap, and switches ON with ultrahigh conductance near the Weyl point due to low-loss topological current. The ON/OFF ratio reaches 108 with ultrahigh ON-state conductance of 39 mS/μm.
Besides, his innovations not only utilize topological states and Weyl-based mechanisms for information transfer but also significantly minimize energy use and reduce heat dissipation. To meet the rising computational demands in AI, Dr Chen has developed neuromorphic transistors using topological material Te. the readout power consumption for neuromorphic computing is as low as fW.
Moreover, MoS2, a key material used in Dr Chen's work, is ideally suited for sensing applications due to its efficient control and low voltage requirements. Its broad operational range further enhances its versatility, making it adaptable to a wide range of AIoT scenarios. Dr Chen shows that MoS2 phototransistor arrays can mimic insect vision by directly detecting motion. A 20×20 array detects movement with 99.2% accuracy using a neural network.
Despite these advancements, Dr Chen acknowledges the challenges of scaling production for commercial use, especially in terms of material stability and prototype fabrication. The need for low-power solutions is urgent as AIoT energy demands rise quickly. While global energy production grows by 10-20% annually, energy use for AI and IoT is doubling every few months. Hence, Dr Chen’s work is a key step toward creating sustainable electronic components for AIoT, supporting the use of clean energy technologies and applications.
低功耗人工智能物聯網的新興運算設備
– 陳杰威博士專訪
應用物理學系研究助理教授
隨著人工智能物聯網(AIoT)對先進感測和運算設備的需求增加,能源消耗成為一大問題。傳統電晶體所使用的電荷訊號載體,其熱力所產生的巨大能源消耗,無法滿足人工智能設備日益增長的能量需求。為此,陳杰威博士正著手開發新一代電晶體,透過拓撲和二維物料的運用,改變電晶體的運行原理的技術帶來根本性改變。
陳博士在新一代拓撲相變電晶體中應用了外爾半導體碲(Te)。以碲製作的電槽能透過調節靜電在不同的拓撲相位之間切換。當費米能階處於能隙中時,低導電率會使電晶體無法通過電流;而接近外爾點時,由於拓撲電流的低損耗特性,超高導電率將啟動電晶體。啟動時的導電率可達39 mS/μm,開關比率甚至能達到108。
這項發明不僅利用拓撲不同形態和外爾機制進行訊號傳輸,還大幅降低能耗和熱量散發。為了迎合人工智能日益增長的運算需求,陳博士採用由碲開發的神經形態電晶體,錄得約fW的低功耗率。
另外,二硫化鉬(MoS2)亦是陳博士用於製作新一代電晶體的核心材料,其高效穩定特性和低電壓需求非常適合感測設備。二硫化鉬的多種特性適用於各種AIoT場景,其光電晶體矩陣結構能模仿昆蟲的視覺,檢測移動物體。在結合神經網絡技術後,20×20矩陣在偵測物體移動時的準確率高達99.2%。
雖然研究取得進展,陳博士也明白將技術推向市場的挑戰,尤其在物料供應和製造原型方面。隨著AIoT對降低能源消耗的需求增加,開發低功耗設備成為核心技術。儘管全球能源產量每年增長10%至20%,但AIoT的能耗每幾個月便會翻倍。因此,陳博士的新一代可持續電晶體為行業提供了新可能,並為清潔能源技術和應用奠定基礎。