Human-Robot Interaction (HRI) and Reinforcement Learning
Distinguished Research Seminar Series
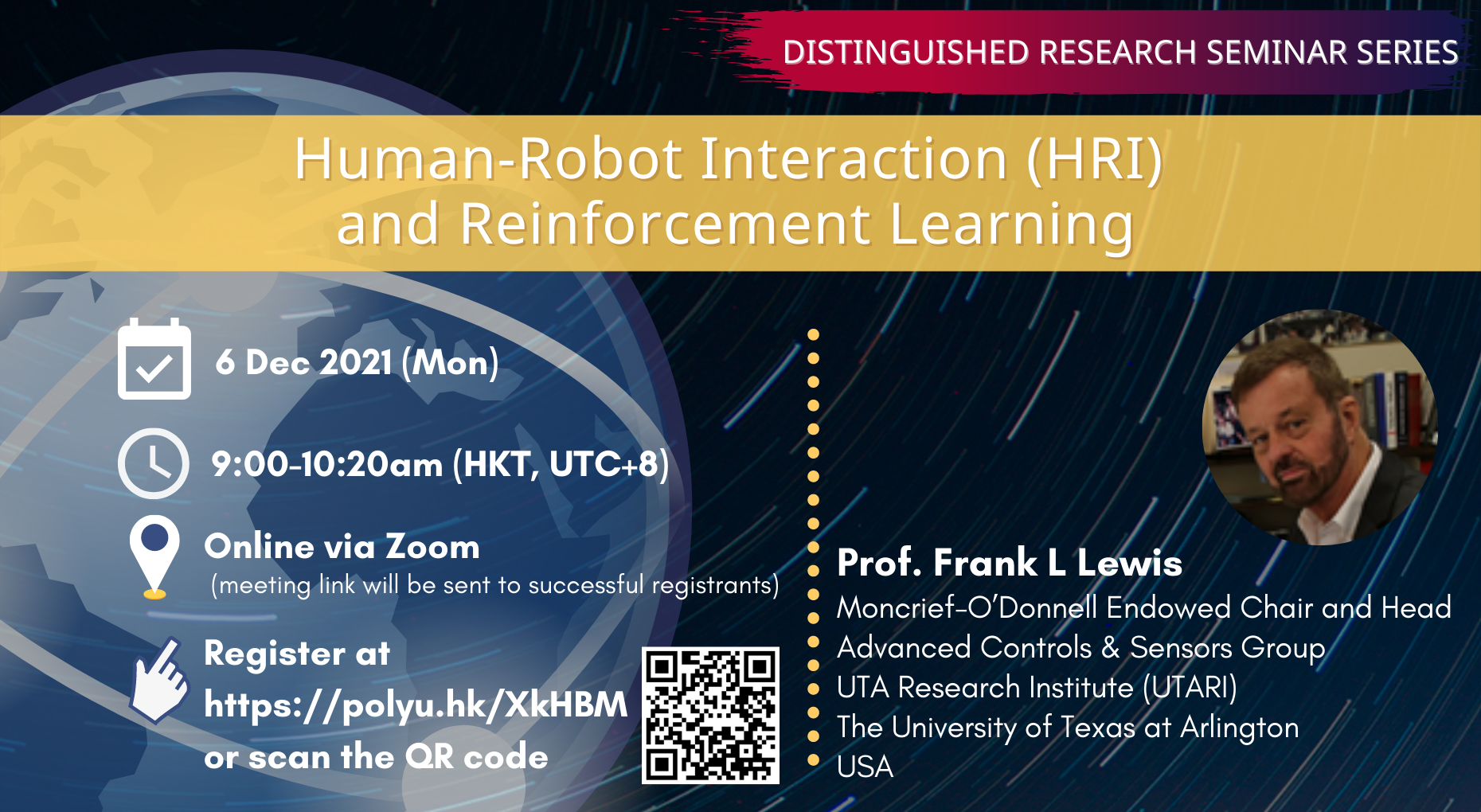
-
Date
06 Dec 2021
-
Organiser
Department of Industrial and Systems Engineering, PolyU
-
Time
09:00 - 10:20
-
Venue
Online via ZOOM
Speaker
Prof. Frank. L. Lewis
Remarks
Meeting link will be sent to successful registrants
Summary
Human-Robot Interaction. Co-robotics involves humans and robots working together safely in the same shared space as a team. This motivates physical Human-Robot Interaction (HRI) systems that adapt to different humans and have guaranteed robustness and stability properties. For modern interactive HRI systems to be capable of performing a wide range of tasks successfully, it is required to include the effects of both the robot dynamics and the human operator dynamics.
Human performance neuropsychological and human factors studies have shown that in coordinated motion with a robot, human learning has two components. The human operator learns a robot-specific inverse dynamics model to compensate for the nonlinearities of the robot, and simultaneously learns a feedback control component that is specific to the successful performance of the task. We incorporate these foundations in the design of HRI control systems by using a new Two Loop HRI Design - an Inner Design Loop to control the robot dynamics and an Outer Design Loop that includes task and human dynamics information. Three adaptive HRI outer loop designs are given that assist the human operator to perform a given task with minimum human workload demands and improved overall human-robot system performance. Experimental results on a PR2 robot demonstrate the effectiveness of this approach in using the robot to improve the human’s performance of a motion task.
Reinforcement Learning. In the second part of the course, techniques from reinforcement learning are used to design a new family of adaptive controllers based on actor-critic mechanisms that converge in real time to optimal control and game theoretic solutions. Using our technique known as Integral Reinforcement Learning (IRL), we will develop Optimal Control reinforcement learning methods for Continuous-Time Systems that do not require knowledge of the system drift dynamics. In the linear quadratic LQR case, the new RL adaptive control algorithms learn the solution to the Riccati equation by adaptation along the system motion trajectories. In the case of nonlinear systems with general performance measures, the algorithms learn the solutions of Hamilton-Jacobi or HJI equations. New IRL algorithms will be presented for solving online several classes of multi-player games. Finally these new IRL techniques are applied to HRI optimal task-loop design
Keynote Speaker
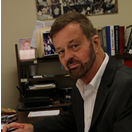
Prof. Frank. L. Lewis
Moncrief-O’Donnell Endowed Chair and Head
Advanced Controls & Sensors Group
UTA Research Institute (UTARI)
The University of Texas at Arlington, USA
F.L. Lewis: Member, National Academy of Inventors. Fellow IEEE, Fellow IFAC, Fellow AAAS, Fellow U.K. Institute of Measurement & Control, PE Texas, U.K. Chartered Engineer. UTA Distinguished Scholar Professor, UTA Distinguished Teaching Professor, and Moncrief-O’Donnell Chair at The University of Texas at Arlington Research Institute. Founding Member Mediterranean Control Association. Ranked at position 89 worldwide, 62 in the USA, and 3 in Texas of all scientists in Computer Science and Electronics, by Guide2Research. Bachelor's Degree in Physics/EE and MSEE at Rice University, MS in Aeronautical Engineering at Univ. W. Florida, Ph.D. at Ga. Tech. He works in feedback control, reinforcement learning, intelligent systems, and distributed control systems. Author of 8 U.S. patents, 420 journal papers, 426 conference papers, 20 books, 48 chapters, and 12 journal special issues. He received the Fulbright Research Award, NSF Research Initiation Grant, ASEE Terman Award, Int. Neural Network Soc. Gabor Award 2009, U.K. Inst. Measurement & Control Honeywell Field Engineering Medal 2009. Received AACC Ragazzini Education Award 2018, IEEE Computational Intelligence Society Neural Networks Pioneer Award 2012 and AIAA Intelligent Systems Award 2016. IEEE Control Systems Society Distinguished Lecturer. Received Outstanding Service Award from Dallas IEEE Section, selected as Engineer of the Year by Ft. Worth IEEE Section. Listed in Ft. Worth Business Press Top 200 Leaders in Manufacturing. Received the 2010 IEEE Region 5 Outstanding Engineering Educator Award and the 2010 UTA Graduate Dean’s Excellence in Doctoral Mentoring Award. Elected to UTA Academy of Distinguished Teachers 2012. Texas Regents Outstanding Teaching Award 2013. He served on the NAE Committee on Space Station in 1995.
You may also like