Novel AI-driven Diagnostics for Heart Diseases
Cardiovascular disease is the major cause of death globally. Two novel systems driven by
advanced AI techniques for non-invasive precise diagnosis for cardiovascular diseases were developed
First, a novel precise yet super-efficient diagnosis system for coronary artery disease
(CAD) was proposed, which is the major cause of around half of the deaths relevant to cardiovascular disease. The
proposed system ramps up the current solutions in two fundamental yet critical aspects.
On the one hand, the accuracy and efficiency of patient-specific anatomical model
reconstruction were improved by developing a novel interactive unsupervised deep learning model for automated
coronary artery segmentation and a data-driven model for mesh optimization.
On the other hand, a novel lattice Boltzmann method (LBM) based solver and a deep
learning model equipped with an active learning strategy were developed to accelerate the computation intensive 3D
FFR map calculation.
The proposed system has potential to benefit numerous CAD patients, minimizing the ratio of
unnecessary invasive testing, improving the patient outcomes, and reducing healthcare cost, and
hence also has significant commercial value.
A novel AI-driven system for the diagnosis of congenital heart diseases (CHD) from
echocardiography was also developed by integrating a set of advanced deep learning models for automating key steps in
the diagnosis procedure, including standard plane acquisition, defect classification, and diagnostic
quality control.
It is believed that the system will not only promote the research of AI-driven medical image analysis but also has great social impact and commercial potential.
advanced AI techniques for non-invasive precise diagnosis for cardiovascular diseases were developed
First, a novel precise yet super-efficient diagnosis system for coronary artery disease
(CAD) was proposed, which is the major cause of around half of the deaths relevant to cardiovascular disease. The
proposed system ramps up the current solutions in two fundamental yet critical aspects.
On the one hand, the accuracy and efficiency of patient-specific anatomical model
reconstruction were improved by developing a novel interactive unsupervised deep learning model for automated
coronary artery segmentation and a data-driven model for mesh optimization.
On the other hand, a novel lattice Boltzmann method (LBM) based solver and a deep
learning model equipped with an active learning strategy were developed to accelerate the computation intensive 3D
FFR map calculation.
The proposed system has potential to benefit numerous CAD patients, minimizing the ratio of
unnecessary invasive testing, improving the patient outcomes, and reducing healthcare cost, and
hence also has significant commercial value.
A novel AI-driven system for the diagnosis of congenital heart diseases (CHD) from
echocardiography was also developed by integrating a set of advanced deep learning models for automating key steps in
the diagnosis procedure, including standard plane acquisition, defect classification, and diagnostic
quality control.
It is believed that the system will not only promote the research of AI-driven medical image analysis but also has great social impact and commercial potential.
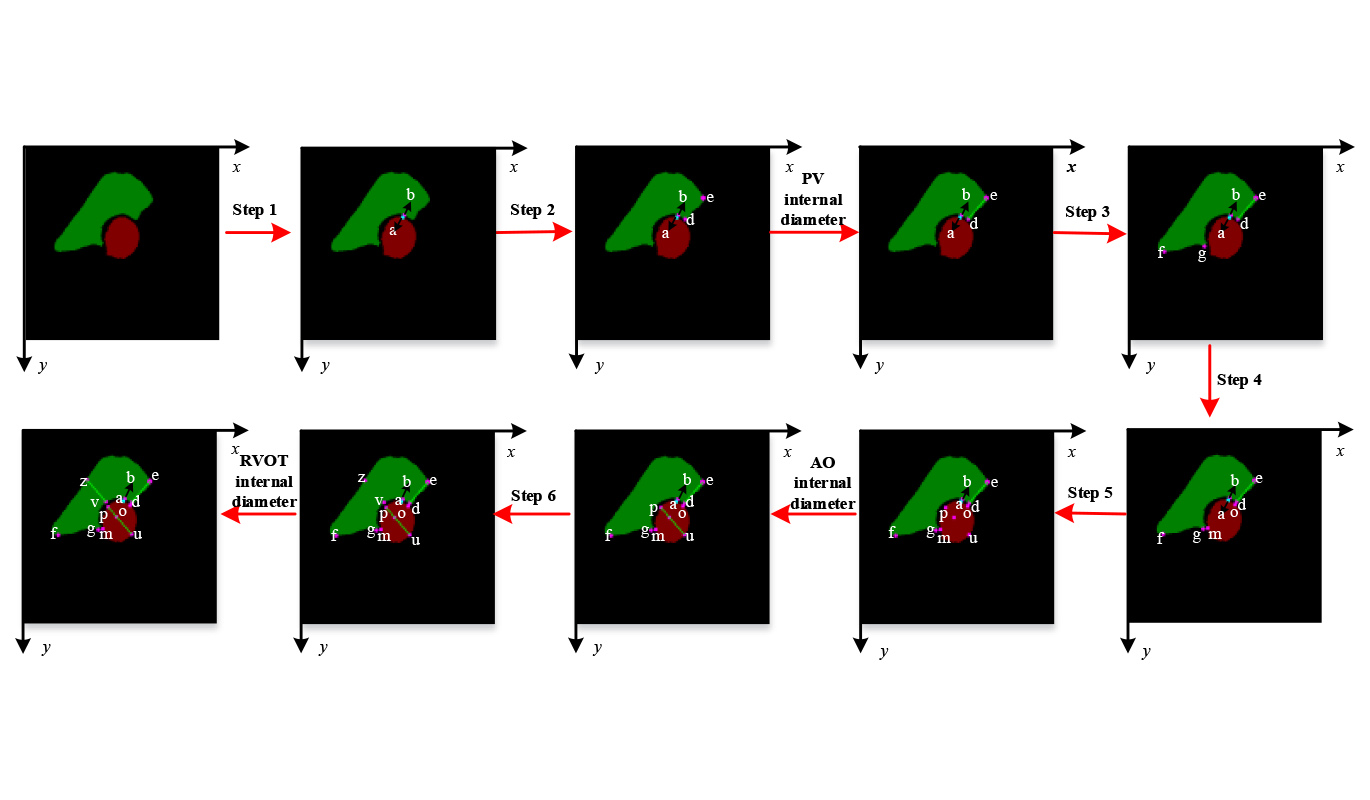
The network architecture of our detection model and detection and measurement results.