Academic Staff
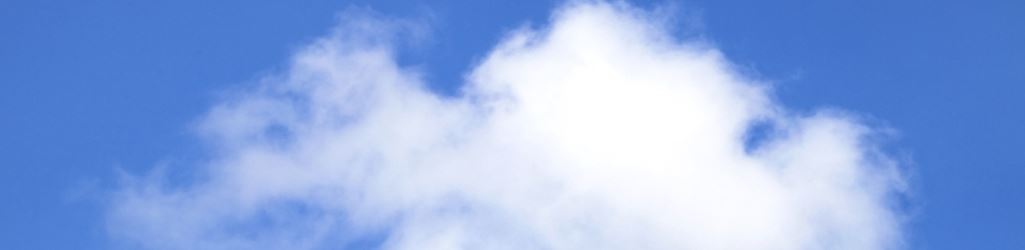
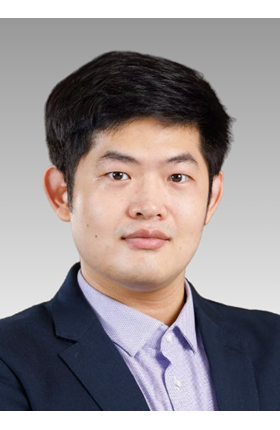
Prof. Siyuan FENG
Assistant Professor
- R811
- 3400 2408
- siyuan.feng@polyu.edu.hk
Biography
Ph.D. (HKUST); M.Sc. (UC Berkeley); B.Eng. (Tongji)
Prof. Siyuan Feng is an Assistant Professor at the Department of Aeronautical and Aviation Engineering, The Hong Kong Polytechnic University (PolyU). Prior to this, he was a Research Assistant Professor at PolyU. Prof. Feng received his PhD degree in Transportation Engineering from The Hong Kong University of Science and Technology in 2023, supported by the Hong Kong PhD Fellowship Scheme. He obtained his MSc degree in Systems Engineering from the University of California, Berkeley in 2019, and his BSc degree in Civil Engineering from Tongji University in 2018. His research focus on the design and development of modelling, machine learning, operation research and simulation methods for solving problems in aviation and transportation systems. His research has attracted funding from Research Grants Council of Hong Kong and industry partners. The research of him has been published in leading international journals, including Transportation Research Part C/E, IEEE Transactions on Intelligent Transportation Systems, etc.
Research Interests
- Machine learning for multi-modal transportation systems
- Low-altitude air mobility and logistics
- Aviation management
- Aviation and transportation simulation
- Shared mobility
Academic Awards
- Young Scientist Awards of Hong Kong Institution of Science, Shortlisted (2024)
- Hong Kong Society for Transportation Studies (HKSTS) Outstanding Dissertation Award,Honorable Mention (2024)
- Best Paper Award, The 15th International Workshop on Computational Transportation Science (CTS 2024)
- HKUST SENG PhD Research Excellence Award, Finalist (2024)
- RedBird Academic Excellence Award (2022)
Opening Positions
Self-motivated engineering, science, and business students with strong academic backgrounds and interests in pursuing a PhD degree in the areas of aviation, low-altitude air mobility, transportation, machine learning and operations research are strongly encouraged to contact me.
Selected Publications
- Chen, T., Shen, Z., Feng, S.*, Yang, L., & Ke, J. (2025). Dynamic matching radius decision model for on-demand ride services: A deep multi-task learning approach. Transportation Research Part E: Logistics and Transportation Review, 193, 103822.
- Wang, J., Feng, S.*, & Yang, H. (2024). Spatial–Temporal Upfront Pricing Under a Mixed Pooling and Non-Pooling Market With Reinforcement Learning. IEEE Transactions on Intelligent Transportation Systems.
- Chen, K., Liang, Y., Han, J., Feng, S.*, Zhu, M., & Yang, H. (2024). Semantic-fused multi-granularity cross-city traffic prediction. Transportation Research Part C: Emerging Technologies, 162, 104604.
- Wei, S., Feng, S.*, Yang, H. (2024) Multi-view spatial-temporal graph convolutional network for traffic prediction. IEEE Transactions on Intelligent Transportation Systems.
- Chen, K., Han, J., Feng, S.*, Zhu, M., & Yang, H. (2023). Region-Aware Hierarchical Graph Contrastive Learning for Ride-Hailing Driver Profiling. Transportation Research Part C: Emerging Technologies, 156, 104325.
- Feng, S., Wei, S., Zhang, J., Li, Y., Ke, J., Chen, G., Zheng, Y., Yang, H (2023). A macro-micro spatio-temporal neural network for traffic prediction. Transportation research part C: emerging technologies, 156, 104331.
- Feng, S., Ke, J., Xiao, F., Yang, H (2022). Approximating a ride-sourcing system with block matching. Transportation Research Part C: Emerging Technologies, 145, 103920.
- Feng, S., Duan, P., Ke, J., and Yang, H. (2022). Coordinating ride-sourcing and public transport services with a reinforcement learning approach. Transportation Research Part C: Emerging Technologies, 138, 103611.
- Ke, J., Feng, S., Zhu, Z., Yang, H., and Ye, J. (2021). Joint predictions of multi-modal ride-hailing demands: A deep multi-task multi-graph learning-based approach. Transportation Research Part C: Emerging Technologies, 127, 103063.
- Feng, S., Ke, J., Yang, H., and Ye, J. (2021). A Multi-Task Matrix Factorized Graph Neural Network for Co-Prediction of Zone-Based and OD-Based Ride-Hailing Demand. IEEE Transactions on Intelligent Transportation Systems. 23(6), 5704 - 5716.
Community services
Reviewer:
- Transportation Research Part C: Emerging Technologies
- Transportation Research Part A: Policy and Practice
- IEEE Transactions on Intelligent Transportation Systems
- IEEE Transactions on Vehicular Technology
- International Journal of Production Economics
- Travel Behaviour and Society
- Journal of Advanced Transportation
- Transportation Research Record