Professor Kenneth Lam
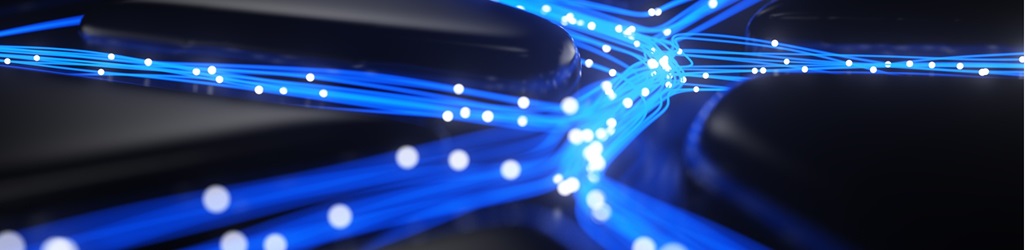
Professor Lam has been actively involved in professional activities. In particular, he was the Secretary of the IEEE ICIP 2010, a Technical Co-Chair of IEEE PCM 2010, and a General Co-Chair of IEEE ICSPCC 2012, APSIPA ASC 2015, and IEEE ICME 2017. He was the Chairman of the IEEE HK Chapter of Signal Processing between 2006 and 2008. He received an Honorable Mention of the Annual Pattern Recognition Society Award for outstanding contribution to the Pattern Recognition Journal in 2004, and a number of best paper awards.
Professor Lam was the Director-Student Services and the Director-Membership Services of the IEEE Signal Processing Society. He was an Associate Editor of IEEE Trans. on Image Processing, and Digital Signal Processing. He was also an Editor of HKIE Transactions, and an Area Editor of the IEEE Signal Processing Magazine. Currently, he is the VP-Publications of the Asia-Pacific Signal and Information Processing Association (APSIPA). He also serves as an Associate Editor of APSIPA Trans. on Signal and Information Processing, and EURASIP Int. Journal on Image and Video Processing. His current research interests include human face recognition, image and video processing, and computer vision.
Research highlights:
More than half of Professor Lam's publications are on face-image analysis and recognition. He has published many good technical papers, with good citations. In particular, he has proposed many different algorithms for tackling the problems of illumination, facial-expression, and pose variations for face recognition, as well as algorithms for face detection, facial landmark localization, facial feature extraction, etc. In recent years, he has focused more on research on low-resolution (LR) face recognition, where he proposed super-resolving facial features, rather than super-resolving the LR query face. By super-resolving facial features directly from the low-resolution query image, the estimated higher-resolution features are more accurate than those extracted from a super-resolved, distorted face image. >He also proposed an approach, which considers face super-resolution and recognition, simultaneously. These two tasks are related and can help each other. Considering them simultaneously can achieve better performances for both face super-resolution and recognition. He also proposed new research for high-resolution face recognition. His proposed idea is totally different from conventional face-recognition methods. Rather than analysing and comparing the facial features between face images for face recognition, he devised a method to detect pores in face images, and matching two faces using pore-scale features. The advantage of the approach is that the pore patterns are unique for different people, and this is sufficient to make an accurate decision by using a small facial skin region. He has also built a data set for learning pore patterns. Recently, he has also proposed various algorithms for age-invariant face recognition. In particular, feature-encoding methods have been applied to further enhance the robustness of deep features.
A large number of the pore patterns from two face images of the same subject can be matched, but only a few pore patterns can be matched for face images of different subjects.