Using Advanced LLMs to Enhance Smaller LLMs: An Interpretable Knowledge Distillation Approach
Research Seminar Series
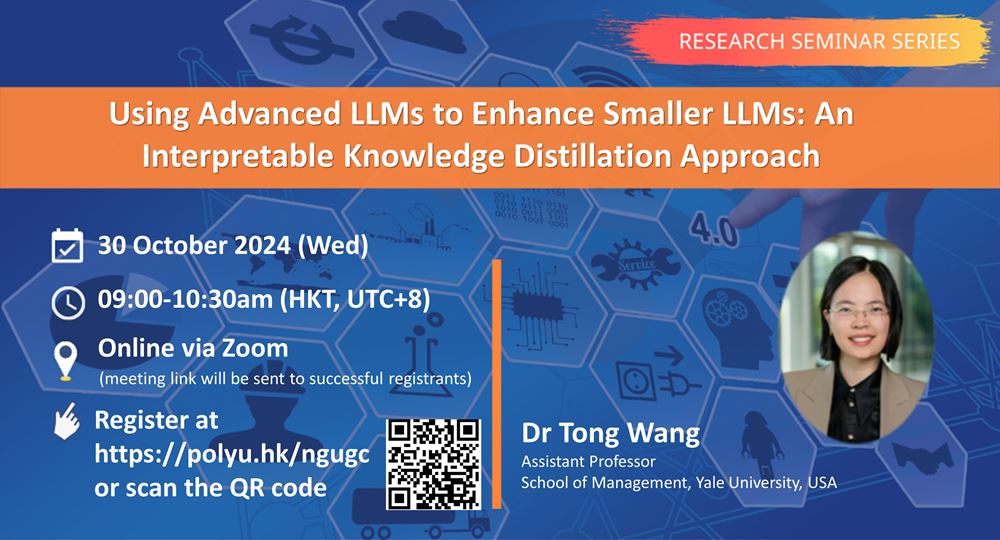
-
Date
30 Oct 2024
-
Organiser
Department of Industrial and Systems Engineering, PolyU
-
Time
09:00 - 10:30
-
Venue
Online via ZOOM
Speaker
Dr Tong Wang
Remarks
Meeting link will be sent to successful registrants
Summary
Large language models (LLMs) like GPT-4 or LlaMa 3 provide superior performance in complex human-like interactions. But they are costly, or too large for edge devices such as smartphones and harder to self-host, leading to security and privacy concerns. This paper introduces a novel interpretable knowledge distillation approach to enhance the performance of smaller, more economical LLMs that firms can self-host. We study this problem in the context of building a customer service agent aimed at achieving high customer satisfaction through goal-oriented dialogues. Unlike traditional knowledge distillation, where the "student" model learns directly from the "teacher" model's responses via fine-tuning, our interpretable "strategy" teaching approach involves the teacher providing strategies to improve the student's performance in various scenarios. This method alternates between a "scenario generation" step and a "strategies for improvement" step, creating a customized library of scenarios and optimized strategies for automated prompting. The method requires only black-box access to both student and teacher models; hence it can be used without manipulating model parameters. In our customer service application, the method improves performance, and the learned strategies are transferable to other LLMs and scenarios beyond the training set. The method's interpretabilty helps safeguard against potential harms through human audit.
Keynote Speaker
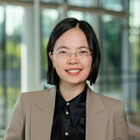
Dr Tong Wang
Assistant Professor
School of Management, Yale University, USA
Tong Wang’s research interests are in developing machine learning solutions for business problems. Her work focuses on creating novel interpretable models that can effectively represent and analyze structured and unstructured data, such as texts and images. The overarching objective of these interpretable models is to extract valuable insights from the data, empowering stakeholders to make well-informed decisions while also facilitating a clear understanding of the decision-making processes employed by the models. Tong received her Ph.D. in Computer Science from Massachusetts Institute of Technology. Prior to joining Yale, she actively pursued research on machine learning solutions for various real-world challenges. Her work on crime pattern detection has been included in Wikipedia Crime Analysis and gained media coverage. The ideas from her algorithm have been implemented by New York Police Department. Tong contributed to the development of an interpretable model for the FICO challenge in 2018, outperforming black-box machine learning models and earning the FICO Recognition Award.
You may also like