Integrating Domain Knowledge and Physics in AI: Harnessing Inductive Bias for Advanced PHM Solutions
Research Seminar Series
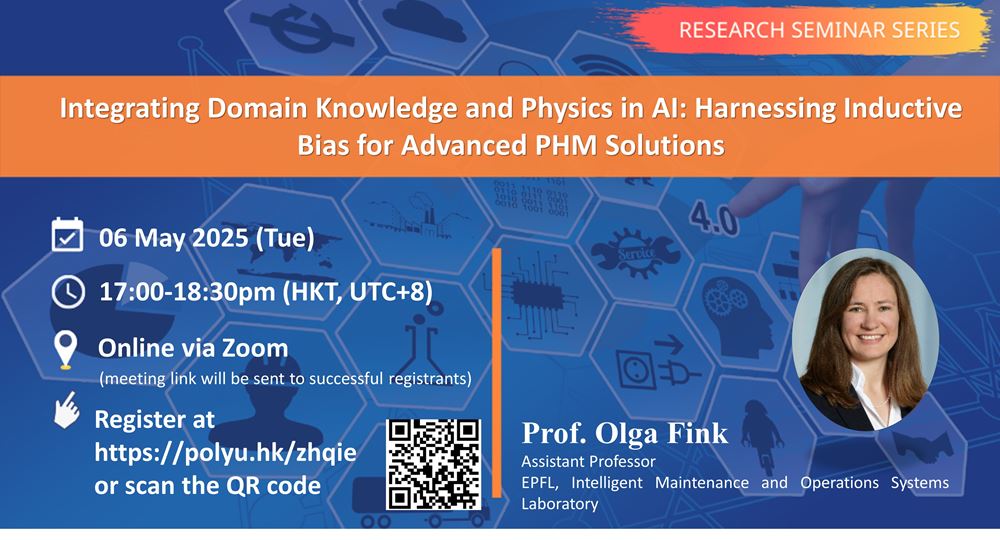
-
Date
06 May 2025
-
Organiser
Department of Industrial and Systems Engineering, PolyU
-
Time
17:00 - 18:30
-
Venue
Online via ZOOM
Speaker
Dr Olga Fink
Remarks
Meeting link will be sent to successful registrants
Summary
In the field of prognostics and health management, the integration of machine learning has enabled the development of advanced predictive models that ensure the reliable and safe operation of complex assets. However, challenges such as sparse, noisy, and incomplete data necessitate the integration of prior knowledge and inductive bias to improve model generalization, interpretability, and robustness.
Inductive bias, defined as the set of assumptions embedded in machine learning models, plays a crucial role in guiding these models to generalize effectively from limited training data to real-world scenarios. In PHM applications, where physical laws and domain-specific knowledge are fundamental, the use of inductive bias can significantly enhance a model’s ability to predict system behavior under diverse operating conditions. By embedding physical principles into learning algorithms, inductive bias reduces the reliance on large datasets, ensures that model predictions are physically consistent, and enhances both the generalizability and interpretability of the models.
This talk will explore various forms of inductive bias tailored for PHM systems, with a particular focus on heterogenous-temporal graph neural networks, as well as physics-informed and algorithm-informed graph neural networks. These approaches will be applied to virtual sensing, modelling multi-body dynamical systems and anomaly detection.
Keynote Speaker
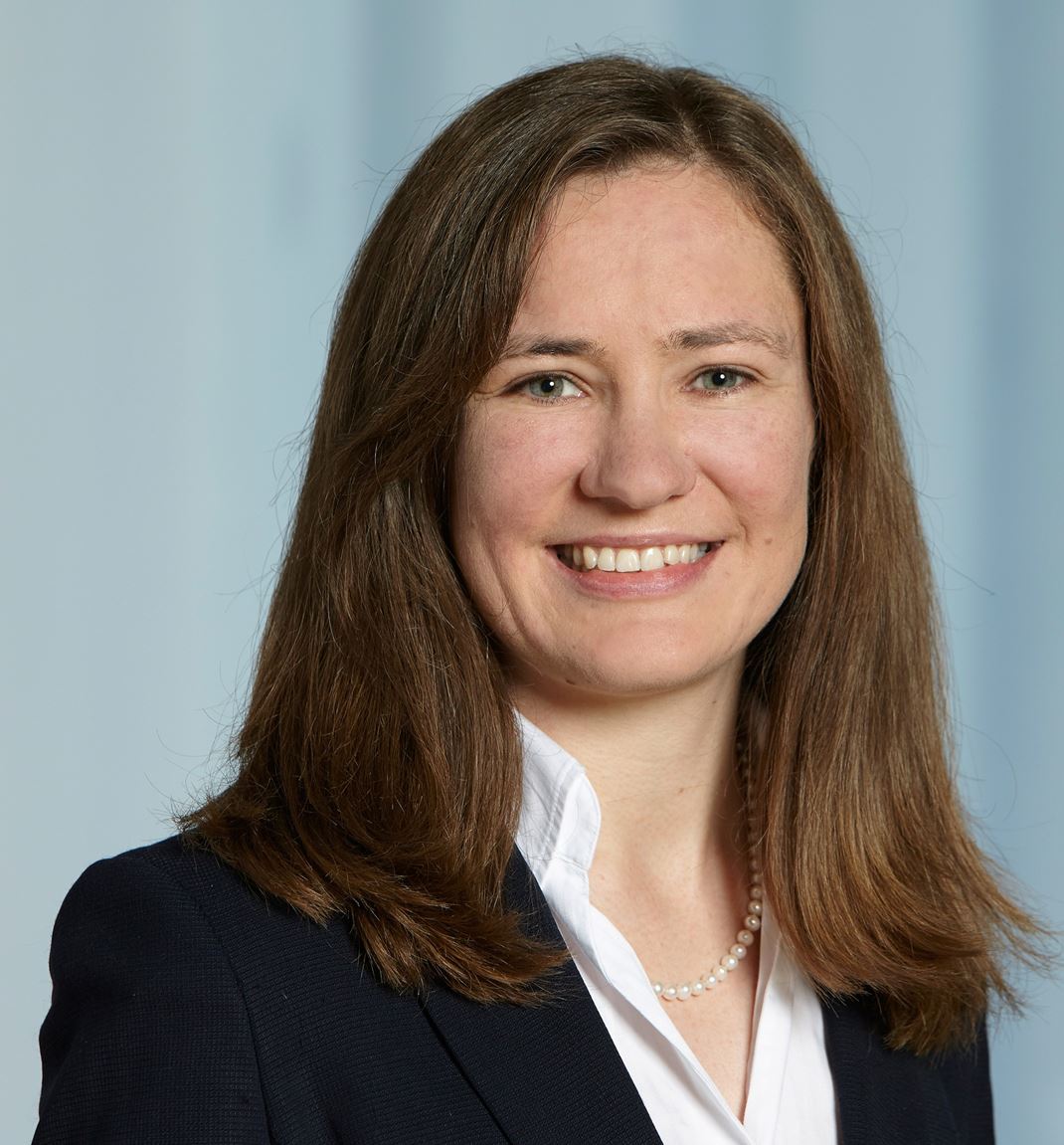
Dr Olga Fink
Assistant Professor
Intelligent Maintenance and Operations Systems Laboratory, EPFL (Swiss Federal Technology Institute of Lausanne), Switzerland
Olga Fink is an assistant professor leading the Laboratory of Intelligent Maintenance and Operations Systems at EPFL since March 2022. Her research focuses on advancing intelligent maintenance and operations of complex infrastructure and industrial assets through the development of Physics-Informed Machine Learning, Graph Neural Networks, Domain Adaptation, Self-Supervised Learning, Deep Reinforcement Learning, and Multi-Agent Systems. Previously, she was an assistant professor at ETH Zurich from 2018 to 2022, where she was awarded the Swiss National Science Foundation (SNSF) Professorship Grant. From 2014 to 2018, she led the “Smart Maintenance” group at Zurich University of Applied Sciences (ZHAW). Olga holds a Ph.D. from ETH Zurich and a Diploma in Industrial Engineering from Hamburg University of Technology. She serves on the editorial boards of several prestigious journals, including Mechanical Systems and Signal Processing, Engineering Applications of Artificial Intelligence, Reliability Engineering and System Safety and IEEE Internet of Things. Olga has received numerous awards and honors including being named one of Switzerland's 'Top 100 Women in Business' in 2018, recognition as a Young Scientist by the World Economic Forum and World Laureate Forum, and being named a Fellow of the Prognostics and Health Management Society in 2023.
You may also like