Making AI Smarter
Training AI models like humans can inspire studies on the human brain.
The amazing power of AI to learn the rules and patterns of human languages has excited many people. But for Professor Li Ping, Dean of the Faculty of Humanities and Sin Wai Kin Foundation Professor in Humanities and Technology, it is even more exciting to find an improved way to train Large Language Models (LLMs) to process language and perform like human brains.
LLMs are AI models pre-trained on a vast amount of data to become capable of generating human-like languages. An example of LLMs is ChatGPT, a chatbot developed by the company OpenAI.
Currently, the pre-training of LLMs mainly relies on contextual word prediction. Similar methods are used to pre-train many generative artificial intelligence (GenAI) platforms, which can generate images, videos and other data apart from text in response to written prompts, to process language. However, word prediction is only one of the ways the human brain deals with language. Humans also integrate high-level information, such as words, sentences, and the larger context of the narrative in natural language to fully comprehend a discourse.
Sentences are better than words
The PolyU research team led by Professor Li Ping investigated the use of next sentence prediction (NSP) in training LLMs. They found that LLMs trained with NSP matched human brain activity in multiple areas much better than those trained only with contextual word prediction. This is because the NSP task required the LLMs to understand the connections between sentences. The improved model with the NSP mechanism also nicely maps onto established neural models of human discourse comprehension.
The results, on the one hand, enable researchers to stimulate LLMs’ discourse comprehension through NSP, helping AI get closer to the human cognitive process. On the other hand, they also give new insights into how human brains process language. For example, scientists can better understand how the brain processes full discourse such as conversations.
Inspiring researchers in AI and neurocognition
Professor Li said, “Our findings suggest that diverse learning tasks such as NSP can improve LLMs to be more human-like, and potentially more efficient like the human brain without needing a massive amount of data. The study can also bring about interactions and collaborations between researchers in AI and neurocognition. This will stimulate future studies on AI-informed brain and brain-inspired AI.”
The study conducted by Professor Li and his team has been published in the academic journal Science Advances.
The recent study on training LLMs led by Professor Li Ping gave insights into brain studies and the development of AI models.
Professor Li Ping
• Dean of the Faculty of Humanities
• Sin Wai Kin Foundation Professor in Humanities and Technology.
Research Centre Founded to Aid AI Model Training
PolyU has established the Centre for Large AI Models (CLAIM) under the Research Centre for Data Science and Artificial Intelligence to meet the high demand for computing resources necessary for training large AI models with the primary objective to provide PolyU researchers with the essential infrastructure to train AI models effectively.
While fostering advancements in AI research and application across art, science, engineering, and other fields, CLAIM will also play a crucial role in promoting the sharing of AI technology within the University.
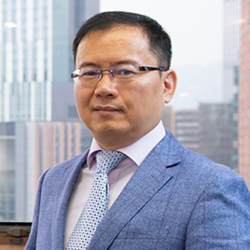
Professor Li Qing
• Co-director of CLAIM
• Chair Professor of Data Science and Head, Department of Computing
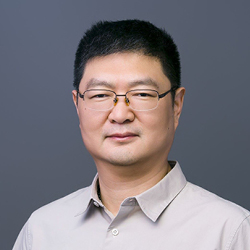
Professor Zhang Lei
• Co-director of CLAIM
• Chair Professor of Computer Vision and Image Analysis, Department of Computing